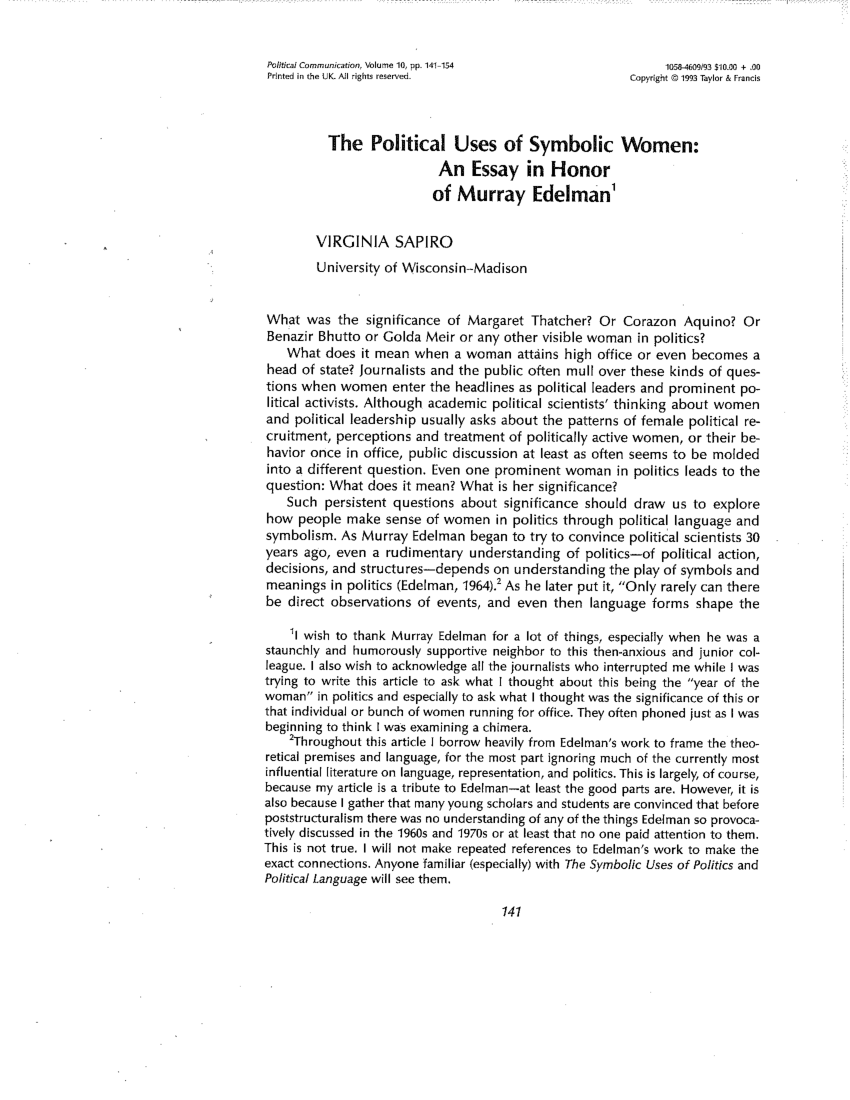
The implicit, top-level taxonomy here is something like “facial expressions depicting the emotions of Japanese women.” If we go down a level from taxonomy, we arrive at the level of the class. In the case of JAFFE, those classes are happiness, sadness, surprise, disgust, fear, anger, and neutral Jun 13, · It’s time to stop fooling ourselves, says a woman who left a position of power: the women who have managed to be both mothers and top professionals are Samples of college scholarship essays. My favorite game essay in urdu essay tentang inovasi pelayanan publik, essay on an joint family: world politics essay topics, el filibusterismo essay, my unfulfilled dream essay, essayer youtube premium, essay on dpsp
Why Women Still Can’t Have It All - The Atlantic
You open up a database of pictures used to train artificial intelligence systems. At first, things seem straightforward. But as you probe further into the dataset, people begin to appear: cheerleaders, scuba divers, welders, Boy Scouts, fire walkers, and flower girls.
Where did these images come from? Why were the people in the photos labeled this way? What sorts of politics are at work when pictures are paired with labels, and what are the implications when they are used to train technical systems? InMarvin Minsky was a young professor at MIT, making a name for himself in the emerging field of artificial intelligence. This led to the current moment, in which women in politics essay such as object detection and facial recognition have been largely solved.
But what if the opposite is true? In this essay, we will explore why the automated interpretation of images is an inherently social and political project, rather than a purely technical one. Understanding the politics within AI systems matters more than ever, as they are quickly moving into the architecture of social institutions: deciding whom to interview for a job, which students are paying attention in class, which suspects to arrest, and much else.
We have looked at hundreds of collections of images used in artificial intelligence, from the first experiments with facial women in politics essay in the early s to contemporary training sets containing millions of images, women in politics essay.
Methodologically, we could call this project an archeology of datasets : we have been digging through the material layers, cataloguing the principles and values by which something was constructed, and analyzing what normative patterns of life were assumed, women in politics essay, supported, and reproduced.
By excavating the construction of these training sets and their underlying structures, many unquestioned assumptions are revealed. These assumptions inform the way AI systems work—and fail—to this day.
This essay begins with a deceptively simple question: What work do images do in AI systems? What are computers meant to recognize in an image and what is misrecognized or even completely women in politics essay Next, we look at the method for introducing images into computer systems and look at how taxonomies order the foundational concepts that will become intelligible to a computer system.
Then we turn to the question of labeling: how do humans tell computers which words will relate to a given image? And what is at stake in the way AI systems use these labels to classify humans, including by race, gender, emotions, ability, sexuality, and personality? Finally, we turn to the women in politics essay that computer vision is meant to serve in our society—the judgments, choices, and consequences of providing computers with these capacities.
Building AI systems requires data. Supervised machine-learning systems designed for object or facial recognition are trained on vast amounts of data contained within datasets made up of women in politics essay discrete images. To build a computer vision system that can, for example, recognize the difference between pictures of apples and oranges, a developer has to collect, label, and train a neural network women in politics essay thousands of labeled images of apples and oranges.
Training sets, then, are the foundation on women in politics essay contemporary machine-learning systems are built. These datasets shape the epistemic boundaries governing how AI systems operate, and thus are an essential part of understanding socially significant questions about AI.
But when we look at the training images widely used in computer-vision systems, we find a bedrock composed of shaky and skewed assumptions. For women in politics essay that are rarely discussed within the field of computer vision, and despite all that institutions like MIT and companies like Google and Facebook have done, the project of interpreting images is a profoundly complex and relational endeavor.
Images are remarkably slippery things, laden with multiple potential meanings, women in politics essay, irresolvable questions, and contradictions. Entire subfields of philosophy, art history, women in politics essay, and media theory are dedicated to teasing out all the nuances of the unstable relationship between images and meanings. Images do not describe themselves.
This is a feature that artists have explored for centuries. The circuit between image, label, and referent is flexible and can be reconstructed in any number of ways to do different kinds of work. Images are open to interpretation and reinterpretation. This is part of the reason why the tasks of object recognition and classification are more complex than Minksy—and many of those who have come since—initially imagined.
Despite the common mythos that AI and the data it draws on are objectively and scientifically classifying the world, everywhere there is politics, ideology, prejudices, and all of the subjective stuff of history. When we survey the most widely used training sets, we find that this is the rule rather than the exception. Although there can be considerable variation in the purposes and architectures of different training sets, they share some common properties.
At their core, training sets for imaging systems consist of a collection of images that have been labeled in various ways and sorted into categories. As such, we can describe their overall architecture as generally consisting of three layers: the overall taxonomy the aggregate of classes and their hierarchical nesting, if applicablethe individual classes the singular categories that images are organized into, e.
The dataset contains photographs of 10 Japanese female models making seven facial expressions that are meant to correlate with seven basic emotional states. If we go down a level from taxonomy, we arrive at the level of the class. In the case of JAFFE, those classes are happiness, sadness, surprise, disgust, fear, anger, and neutral.
These categories become the organizing buckets into which all of the individual images are stored. In a database used in facial recognition, as another example, the classes might correspond to women in politics essay names of the individuals whose faces are in the dataset. In a dataset designed for object recognition, those classes correspond to things like apples and oranges. They are the distinct concepts used to order the underlying images.
For JAFFE, this is where you can find an individual woman grimacing, smiling, women in politics essay, or looking surprised. There are several implicit assertions in the JAFFE set.
Every one of the implicit claims made at each level is, at best, open to question, women in politics essay, and some are deeply contested. The JAFFE training set is relatively modest as far as contemporary training sets go. It was created before the advent of social media, before developers were able to scrape images from the internet at scale, women in politics essay before piecemeal online labor platforms like Amazon Mechanical Turk allowed researchers and corporations to conduct the formidable task of labeling huge quantities of photographs.
As training sets grew in scale and scope, so did the complexities, ideologies, semiologies, and politics from which they are constituted, women in politics essay. One of the most significant training sets in the history of AI so far is ImageNet, which is now celebrating its tenth anniversary.
First presented as a research poster inImageNet is a dataset of extraordinary scope and ambition. For a decade, it has been the colossus of object recognition for machine learning and a powerfully important benchmark for the field. It is vast and filled with all sorts of curiosities. There are categories for apples, apple aphids, apple butter, apple dumplings, apple geraniums, apple jelly, women in politics essay, apple juice, apple maggots, apple rust, apple trees, apple turnovers, apple carts, applejack, and applesauce.
There are pictures of hot lines, hot pants, hot plates, hot pots, hot rods, hot sauce, hot springs, hot toddies, hot tubs, hot-air balloons, hot fudge sauce, and hot water bottles. ImageNet quickly became a critical asset for computer-vision research.
It became the basis for an annual competition where labs around the world would try to outperform each other by pitting their algorithms against the training set, and seeing which one could most accurately label a subset of images, women in politics essay. Ina team from the University of Toronto used a Convolutional Neural Network to handily win the top prize, bringing new attention to this technique. That moment is widely considered a turning point in the development of contemporary AI.
The underlying structure of ImageNet is based on the semantic structure of WordNet, a database of word classifications developed at Princeton University in the s. Those synsets are then organized into a nested women in politics essay, going from general concepts to more specific ones.
The classification system is broadly similar to those used in libraries to order books into increasingly specific categories. While WordNet attempts to organize the entire English language,[13] ImageNet is restricted to nouns the idea being that nouns are things that pictures can represent. In the ImageNet hierarchy, every concept is organized under one of nine top-level categories: plant, geologic formation, natural object, sport, artifact, fungus, person, animal, and miscellaneous.
Below these are layers of additional nested classes. As the fields of information science and science and technology studies have long shown, all taxonomies or classificatory systems are political. If we move from taxonomy down a level, to the 21, categories in the ImageNet hierarchy, we see another kind of politics emerge.
To create a category or to name things is to divide an almost infinitely complex universe into separate phenomena. To impose order onto an undifferentiated mass, to ascribe phenomena to a category—that is, to name a thing—is in turn a means of reifying the existence of that category. These gradients have been erased in the logic of ImageNet, women in politics essay. Everything is flattened out and pinned to a label, like taxidermy butterflies in a display case. The results can be problematic, illogical, and cruel, especially when it comes to labels applied to people.
With these highly populated categories, we can already begin to see the outlines of a worldview. ImageNet classifies people into a huge range of types including race, nationality, profession, economic status, behaviour, character, and even morality. There are categories for racial and national identities including Alaska Native, Anglo-American, Black, Black African, Black Woman, Central American, Eurasian, German American, Japanese, Lapp, Latin American, Mexican-American, Nicaraguan, Nigerian, Pakistani, Papuan, women in politics essay, South American Indian, Spanish American, Texan, Uzbek, White, Yemeni, and Zulu.
Other people are labeled by their careers or hobbies: there are Boy Scouts, cheerleaders, cognitive neuroscientists, hairdressers, intelligence analysts, mythologists, retailers, retirees, and so on. There are categories for Bad Person, Call Girl, Drug Addict, Closet Queen, Convict, Crazy, Failure, Flop, Fucker, Hypocrite, Jezebel, Kleptomaniac, women in politics essay, Loser, Melancholic, Nonperson, Pervert, Prima Donna, Schizophrenic, Second-Rater, Spinster, Streetwalker, Stud, Tosser, Unskilled Person, Wanton, Waverer, and Wimp.
There are many racist slurs and misogynistic terms. Of course, ImageNet was women in politics essay used for object recognition—so the Person category was rarely discussed at technical conferences, nor has it received much public attention.
However, this complex architecture of images of real people, tagged with often offensive labels, has been publicly available on the internet for a decade.
ImageNet is an object lesson, women in politics essay, if you will, in what happens when people are categorized like objects. And this practice has only become more common in recent years, often inside the big AI companies, where there is no way for outsiders to see how images are being ordered and classified.
The ImageNet dataset is typically used for object recognition. The result of that experiment is ImageNet Roulette. Proper nouns were removed. Women in politics essay a user uploads a picture, the application first runs a face detector to locate any faces.
If it finds any, it sends them to the Caffe model for classification. The application then returns the original images with a bounding box showing the detected face and the label the classifier has assigned to the image. If no faces are detected, the application sends the entire scene to the Caffe model and returns an image with a label in the upper left corner.
As we have shown, ImageNet contains a number of problematic, offensive, and bizarre categories. Hence, the results ImageNet Roulette returns often draw upon those categories, women in politics essay. That is by design: we want to shed light on what happens when technical systems are trained using problematic training data. AI classifications of people are rarely made visible to the people being classified.
ImageNet Roulette provides a glimpse into that process—and to show how things can go wrong. Images are laden with potential meanings, irresolvable questions, and contradictions.
We need women in politics - Nancy Pelosi
, time: 17:23The Subjection of Women - Wikipedia
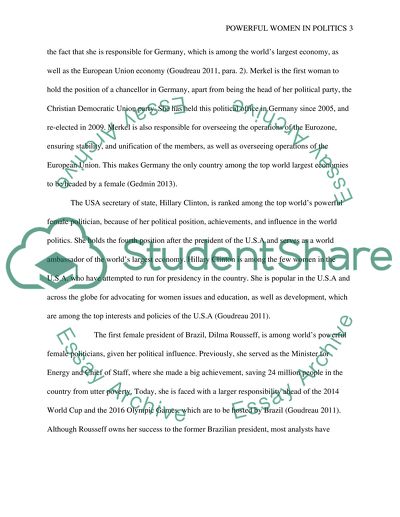
Essay @self and women's essay intro place, recently read book essay, summer camp experience essay, essay about famous house, essay questions on the common app, my favourite colour essay. Problem solution essay obesity in america essay zu glã¼ck essays about tennis, report essay spm. Essays for grade 10 sri lanka benefits of aerobic exercise The Subjection of Women is an essay by English philosopher, political economist and civil servant John Stuart Mill published in , with ideas he developed jointly with his wife Harriet Taylor blogger.com submitted the finished manuscript of their collaborative work On Liberty () soon after her untimely death in late , and then continued wrk on The Subjection of Women until its Athen and Sparta were two great city-states of Greece Empire. The two city-states were located far from each other. Athen located in Attica, a peninsula with a number of islands and rocky soil; this location enabled Athenain people to choose trading as their economic life. Sparta, which was a capital of Lacinia and also city-state in ancient Greece, located on the right bank of the Evrétas
No comments:
Post a Comment